The Coronacceleration: A deepening mistrust in experts as we live through the five stages of model grief
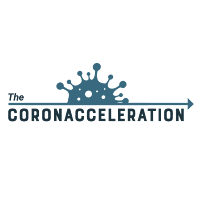
This post was also published on LinkedIn Pulse.
A series on the latent trends that have been accelerated by the Coronavirus
Experts and scientists use hypotheses and models to define their outlook and predictions, which usually are developed, tested and refined over long periods of time. Models can have profound policy implications far beyond experts changing their opinion from don’t wear masks and then to wear masks. With the Cononavirus, we are seeing an unprecedented acceleration of hypotheses and models changing in near real time, leaving heads spinning.The initial Coronavirus models overestimated the death rate by underestimating asymptomatic carriers, and also overestimated the hospitalization rate. The moment that it was clear there was a flawed model was when New York said they needed 30,000 more ventilators and the Federal government said they didn’t. Epidemiologists are scientists, and scientists work with models that are constantly refined. The Governor of New York later said he was working with the Federal government’s model, but clearly the Federal government had used the updated IMHE model, which to the IHME’s credit has been constantly tuned with new data.
Many business people also use models, and there are distinct phases that happen when people start to recognize that a model is inaccurate, which I like to call the “Five stages of model grief.”
Stage 1 - Challenge
Initially, the Coronavirus models used educated guesses based on past coronaviruses as to how many asymptomatic carriers there were using previous Coronaviruses as the baseline. Various serology tests showed that there is likely a vast undercount of people that caught COVID-19 but displayed no symptoms. Many of these studies are aggressively questioned as not perfect, but they are far better data than the educated guess with which the models started. After much challenging of Sweden’s strategy to maintain a limited lockdown in order to achieve immunity in their younger population, the WHO and the press are coming around based on the reality that the death rate in Sweden is similar to other countries such as Ireland, although larger than neighboring countries that will have outbreaks in waves.
Stage 2 - Switch
A common reaction when a model becomes inaccurate is to switch the tracked metric. Initially, there was a focus on the number of projected deaths and hospital surge. When the projected deaths and hospital surge did not happen as predicted in Florida and Georgia, and the curve was over-flattened to only 30% of ICU capacity in California, the metric to track was changed to predicted infection rate. Now, with states and countries re-opening, we should be tracking infection rates rather than death or hospitalization rates.
Stage 3 - Boost
Another common reaction is to boost other variables. With the serology testing beginning to show that there are far more asymptomatic carriers than previously assumed, it significantly lowers the fatality rate of the Coronavirus. Previously there had been numerous reports of an overstated death count, which pivoted to articles boosting the death count without accounting for the fact that numerous people have avoided medical treatment in a time of intense stress.
Stage 4 - Distract
Another common reaction is to bring up ancillary outcomes that are not yet measured, in this case strokes and heart disease, without any metrics as to the occurrence level. “Several” strokes in New York City, a city of over eight million with an estimated 25% infection rate, means that the chance of getting a stroke is 0.00025%. The chance of getting hit by a lightning strike is 0.00014%. Now of course it is fine to discuss ancillary outcomes, however there should be an effort to estimate the chances of those outcomes as well as note that the flu can also cause strokes.
Stage 5 - Deny
Then there is of course the non-reaction to stick with the original narrative no matter what. For example, the head of UCSF epidemiology “doing math” where he continues to use the older, highly questioned case fatality rate and maintains that an infectious respiratory illness will reach 100% of a population, which has never happened.
“Tech Twitter” saw the beginning of an exponential growth curve and thought that it would go up forever, just like they hope their apps will grow. In the end, viral exponential curves, whether in appstore or biological form, inevitably turn into an S, the only thing that is debatable is at what point the top of the S flattens out. Elon Musk, always the outlier, is pointing out that Silicon Valley is sanctimonious and then followed up with a chart of how the models in California have shifted even with social distancing included in the models.
As they say, you can fool some of the people some of the time, but you can’t fool all of the people all of the time. On the other side of the Coronavirus, distrust in experts will increase. This will unfortunately not bode well for well researched climate change models.
“Tech Twitter” saw the beginning of an exponential growth curve and thought that it would go up forever, just like they hope their apps will grow. In the end, viral exponential curves, whether in appstore or biological form, inevitably turn into an S, the only thing that is debatable is at what point the top of the S flattens out. Elon Musk, always the outlier, is pointing out that Silicon Valley is sanctimonious and then followed up with a chart of how the models in California have shifted even with social distancing included in the models.
As they say, you can fool some of the people some of the time, but you can’t fool all of the people all of the time. On the other side of the Coronavirus, distrust in experts will increase. This will unfortunately not bode well for well researched climate change models.